Abstract
Introduction: Rift Valley fever (RVF) represents a significant public health challenge and economic burden due to its impact on livestock and potential to affect humans. Despite attempts to develop vaccines against the Rift Valley fever virus (RVFV), existing options are limited by concerns regarding the inability to differentiate between vaccinated and infected animals, vaccine-associated viremia, and the need for booster doses. This underscores the urgent need for a novel, effective, and safe vaccine, especially for use in ruminants, which this study seeks to address.
Methods: Employing reverse vaccinology—a cutting-edge approach combining bioinformatics and reverse pharmacology—we aimed to develop a novel RVFV vaccine. We focused on the M-glycoprotein segment, identifying highly conserved and immunodominant epitopes in viral glycoprotein sequences from cattle, sheep, and goats in RVF-endemic regions of Africa. Predictions for B- and T-cell epitopes were made, followed by the design of an epitope-based vaccine incorporating ideal linkers and a Bos taurus-specific beta-defensin to enhance immunogenicity. The vaccine's secondary and tertiary structures were analyzed using SOPMA and AlphaFold2, respectively.
Results: The vaccine candidate demonstrated promising physicochemical properties, with the M-glycoprotein sequences showing high antigenicity. Structural analysis revealed a composition of 31.55% alpha helices, 44.92% random coils, 5.35% beta turns, and 18.18% extended strands. Molecular docking with Toll-like receptors 7 and 8 indicated favorable molecular binding interactions, suggesting potential efficacy in stimulating an immune response.
Conclusion: This study paves the way for the development of a novel, safe RVFV vaccine. While the results are promising, further translational research is necessary to confirm the vaccine's effectiveness in animals and its applicability for improving public health outcomes.
Introduction
Rift Valley fever (RVF) is a viral disease most commonly seen in domesticated animals. People can develop RVF through contact with the blood, body fluids, or tissues of infected animals or through bites from infected mosquitoes. Rift Valley fever virus (RVFV) can cause severe disease in newborn ruminants such as sheep, goats, camels, and cattle, with up to a 100% fatality rate. Mosquitoes are the main infection vectors, although sandflies have also shown the capability to transmit the virus1. However, adults seem less susceptible. In ruminants, the virus causes RVF, an acute hemorrhagic fever, accompanied by abortion storms in pregnant females2. Abortion rates may be up to 100% in pregnant animals3. These dire statistics indicate the need for concerted efforts to develop preventive measures against RVFV’s future resurgence.
The RVFV genome is tri-segmented, with large (L), medium (M), and small (S) segments. The L segment encodes the RNA polymerase (RNA-dependent L protein), the S segment encodes the nucleoprotein and the non-structural nucleoprotein (NSs), and the M segment encodes four proteins (two structural glycoproteins [Gn and Gc] and two non-structural glycoproteins [NSm and LGp])4.
RVFV is a vector-borne, zoonotic, negative-stranded, tri-segmented RNA virus that belongs to the genus Phlebovirus and family Bunyaviridae. Apart from affecting human and animal health, it has a negative socio-economic impact5, 6. RVFV has been reported to be endemic to the Arabian Peninsula and Africa1, 7, although it was first identified in Kenya’s Rift Valley in early 19305 (hence its name). Kenya is reported to have experienced the deadliest epizoootic of RVFV before a major outbreak in South Africa between 1950 and 1951, which led to about 500,000 abortions and 100,000 deaths among sheep; subsequent epizootics were observed in Zambia, Namibia, Mozambique, Sudan, Zimbabwe, and other East African countries2, 3. RVFV outbreaks have been associated with periods of greater than average rainfall, although this cannot easily be predicted, thereby suggesting an interplay between the environment and human and animal health3, 8.
Conventional vaccines used extensively for controlling RVF include inactivated, live attenuated vaccines DNA vaccines, viral vector-based vaccines, viral replicon vaccines, and subunit vaccines, among others9. However, these vaccines lack important properties, such as the ability to differentiate infected from vaccinated animals. Live attenuated vaccines induce long-lasting immunity and are generally inexpensive to produce but are linked to an increase in the rate of abortion in pregnant animals and can have negative effects on internal organs, especially the liver, when the attenuated virus spreads inside hepatic cells, as in a naturally occurring RVFV infection9. Other vaccines also face certain difficulties, such as the inability to distinguish between infected and vaccinated animals, viremia, and the requirement for booster shots.
Multi-epitope subunit vaccines incorporate predicted immunodominant epitope peptides from various immunogenic genes that have been identified through proteomic research10. Short immunogenic peptide fragments employed in multi-epitope vaccinations produce potent immune responses while considerably reducing the risk of allergic reactions in the host. Identifying the immunogenic epitopes obtained from viral glycoprotein or nucleocapsid sequences has greatly benefited the in silico design of peptide vaccines11. These epitopes are analyzed and approved using computational techniques based on their physicochemical characteristics, immunogenicity, and non-allergenicity. Subunit vaccines are cost-effective, non-toxic, and safer for animals than other RVFV vaccines. The aforementioned limitations of conventional vaccines and the untapped potential of multi-peptide-based vaccines have made this study necessary.
In this research, we use a computational design approach to develop an RVFV multi-epitope subunit vaccine, which incorporates several antigenic determinants that are conserved across different RVFV strains. The vaccine design is optimized for increased stability, immunogenicity, and manufacturability, using various adjuvants and carrier proteins and evaluated in different delivery systems. Overall, this manuscript describes a comprehensive and innovative approach to the development of an RVFV multi-epitope subunit vaccine, highlighting the potential of this approach for the development of potent vaccines against other viral diseases. Therefore, our objective in this study was to design a safe, non-allergenic, and non-toxic subunit vaccine that can elicit optimal immunity against RVF in ruminants.
Methods
Retrieval of RVF Protein Sequences
The National Center for Biotechnology Information’s (NCBI) immunoinformatics investigation yielded a total of 60 RVFV amino acid sequences, which included sequences from the L, M, and S genomic segments. These sequences came from nations like Kenya, Madagascar, South Africa, Rwanda, Namibia, Egypt, Zimbabwe, and Nigeria.
Antigenicity Prediction
The VaxiJen v2.0 server was used to determine the antigenicity of the RVF proteins11. Without using alignment, the VaxiJen server estimates a protein’s antigenicity based on its physicochemical characteristics. The default threshold value was set at 0.4.
Membrane Topology of Selected Proteins
The trans-membrane topology of the protein sequences was assessed for their intra-cytoplasmic, extra-cytoplasmic, and transmembrane portions using the TMHMM server v2.012. TMHMM predicts transmembrane topology through a hidden Markov model. Proteins with large extra-cytoplasmic regions were considered for further analyses.
Multiple Sequence Alignment
The glycoprotein M sequences underwent repeated sequence alignment using the MEGA 11 program to show conserved regions across the different sequences13. The MUSCLE algorithm was specifically used to align the different protein sequences14.
Prediction of Linear B-Cells
The ABCpred, SVMTriP, and BepiPred servers were used to predict the linear B-cell epitopes. For linear B-cell prediction, ABCpred employs a partly recurrent neural network (Jordan network) with a single hidden layer. The networks comprise 35 residues in a single hidden layer and a changeable optional window length15. SVMTriP is used to locate linear epitopes based on amino acid features such as secondary structure, flexibility, antigenicity, hydrophilicity, and solvent accessibility to predict B-cells16. Using a hidden Markov model, BepiPred forecasts B-cell epitopes from antigen sequences17.
Physicochemical Property Analysis
Analyzing the physicochemical characteristics of the chosen B-cells allowed for evaluating their suitability for vaccine development. ExPASy Protparam was used for this purpose18. Additionally, utilizing VaxiJen, AllerTop, and ToxinPred, these peptides were tested for their allergenicity, antigenicity, and toxicity, respectively11, 19, 20.
Prediction of Cytotoxic T Lymphocytes (CTLs)
The glycoprotein’s (M segment) CTL epitope was predicted using NetMHCII PAN 4.121. The server employs artificial neural networks (ANNs) to forecast peptide binding to MHC alleles. The network is taught to examine peptide ligands that have been eluted by mass spectrometry and have a high binding affinity (BA). The Bovine Leukocyte Antigen (BoLA) was chosen as the reference allele set in this study. The affinity of the glycoprotein peptides for the 20 BoLA alleles BoLA-JSP.1, BoLA-HD6, BoLA-T2c, BoLA-T2b, BoLA-T2a, BoLA-T7, BoLA-D18.4, BoLA-AW10, BoLA-T5, BoLA-1:00901, and BoLA-1:00902 was assessed. The chosen peptide has a length of nine amino acids.
Prediction of Helper T Lymphocytes (HTLs)
Strongly binding MHCII HTL epitopes were predicted using NetMHCII PAN 4.021. Based on machine learning techniques trained on binding affinity or mass spectrometry (eluted ligands) techniques, this tool forecasts the likelihood that a peptide will present an antigen. NETMHCII PAN 4.0 was used to predict epitopes22. The HTL epitopes were chosen to have a default length of 15 amino acids, and the binding affinity of overlapping glycoprotein peptides to the following alleles was discovered (the following DRB1 codes are available): DRB1_0101, DRB1_0102, DRB1_0103, DRB1_0104, DRB1_0105, DRB1_0106, DRB1_0107, DRB1_0108, DRB1_0109, DRB1_0110, DRB1_0111, DRB1_0112, DRB1_0113, DRB1_0115, and DRB1_0116.
Prediction of Interleukin-4- and Interleukin-10-Inducing Epitopes
HTLs, which produce cytokines like interleukins, are crucial in coordinating the immune response. Therefore, using IL4Pred and IL10Pred, the interleukin-4- and interleukin-10-producing capacity of a subset of strongly binding epitopes was evaluated23, 24.
Antigenicity, Toxicity, and Allergenicity Prediction
The toxicity, antigenicity, and allergenicity of strong CTL peptides and HTL epitopes that induce IL-4 and IL-10 were examined. The ToxinPred server, according to Gupta et al.19, predicts peptide toxicity along with its physical properties, such as molecular weight, charge, hydrophobicity, and amphipathicity. The AllerTop v2.0 server reportedly uses machine learning methods including auto- and cross-variance transformation and amino acid E-descriptors and predicts the allergenicity of proteins and peptides, according to Dimitrov et al.20. Doytchinova et al.11 claim that the categorization of antigens by VaxiJen v2.0 is solely based on the physicochemical characteristics of the proteins involved.
Epitope Conservation Determination
B-cells, HTLs, and CTLs were compared via multiple sequence alignment to determine whether they fall within conserved regions. For the final vaccine design, only completely conserved epitopes were considered. For the vaccine to be efficacious across a broad spectrum, highly conserved epitopes are required25. Furthermore, epitopes in the extra-cytoplasmic portion of the glycoprotein sequence were prioritized for selection.
Primary Construct Assembly
The chosen HTL, CTL, and B-cell epitopes served as the building blocks for the vaccine. To increase the final construct’s immunogenicity, a stiff EAAAK linker was used to introduce a Bos taurus-specific beta defense adjuvant to the N-terminus of the vaccine. AAY linkers were used to connect additional CTL epitopes. The HTL and B-cell epitopes were joined together using GPGPG linkers. According to Kavoosi et al.26, the glycine-rich linker GPGPG serves to increase solubility and promote free movement across adjacent domains. To boost the immunogenicity of the vaccine design using the EAAAK linker, a Bos taurus-specific beta defensin adjuvant was also inserted at the N-terminus27.
The Proposed Vaccine’s Allergenicity, Solubility, and Antigenicity
The allergenicity of the vaccine construct was assessed using the AllerTop v.2.0 server, while the antigenicity and solubility of the vaccine construct were assessed using VaxiJen v2.0 and Protein Sol, respectively11, 20, 28.
Analysis of the Vaccine Construct’s Physicochemical Characteristics
Secondary and Tertiary Structure Prediction
The secondary structure was predicted using the SOPMA server. The server predicts protein secondary structure from amino acid sequences using the self-optimized prediction approach29.
The AlphaFold server was used to predict the tertiary structure of the vaccine construct30. AlphaFold uses innovative neural networks in combination with the primary amino acid sequence and alignment sequence of homologs to predict the tertiary structure of a particular protein.
Conformational Epitope Prediction from 3D Vaccines
Thornton’s approach and a residue clustering algorithm on the IEDB database are both used by the ElliPro server to predict conformational epitopes on the 3D structure. According to Ponomarenko et al.31, ElliPro offers a framework for predicting tertiary structure models from amino sequences.
Refinement and Validation of the Tertiary Structure
The quality of the 3D structure of the chosen model, protein structure refinement, protein interaction prediction, GPCR applications, and water position prediction were all improved using the GalaxyWEB refine server32. By recreating and repackaging protein side chains using dynamic simulation techniques, this server improves 3D protein architectures, facilitating the production of proteins with appropriate structural relaxation.
Thereafter, structural analysis using Ramachandran and Z-score analysis was used to validate the vaccine’s structure33, 34. PROCHECK and ProSA-web were used. The Ramachandran plot, which displays the proportion of the amino acids present in the preferred, permitted, and forbidden zones, is produced by the PROCHECK server. ProSA-web performs mathematical analysis on the entire protein structure quality score and presents values and energy.
Codon Optimization and In Silico Cloning
To adapt the vaccine for expression in the Escherichia coli strain K-12 sub-strain MG1655 chosen as the expression host, codon optimization was performed using JCAT35. This is crucial for maximizing protein expression during the recombinant vaccine’s cloning process. Using the SnapGene v5.1.7 program, the chimeric model was virtually cloned into an appropriate expression vector, E. coli K12 pET-28a (+)36.
Molecular Docking
A computational procedure called molecular docking is used to predict the affinities of the ligand and receptor to create stable complexes. Using the HDOCK server, the final vaccine design was docked against toll-like receptor (TLR) 7 and 837.
Genome Segment | Protein | Antigenicity |
---|---|---|
L | Polymerase | Non-antigen |
M | Glycoprotein | Antigen |
Nucleocapsid | Non-antigen |
Genome Segment | Protein | Protein Segment | Position |
---|---|---|---|
L | Polymerase | 1-2091 | Inside |
M | Glycoprotein | 1-16 | Signal peptide |
17-584 | Outside | ||
585-603 | TMHelix | ||
604-675 | Inside | ||
676-685 | TMHelix | ||
686-1159 | Outside | ||
1160-1178 | TMHelix | ||
1179-1197 | Inside | ||
Nonstructural | 1-265 | Inside | |
Nucleocapsid | 1-245 | Inside |
Peptide | Antigenicity | Allergen city |
---|---|---|
DSLREEEMPE | Antigenic | Non- allergic |
VYLDKLDLKTEENLLPD | Antigenic | Non- allergic |
SYWTGSFSPKCLSSRRCHLV | Antigenic | Non- allergic |
SKLKTKMKGVCEVGVQALKK | Antigenic | Non- allergic |
Peptides | Antigenicity score | Instability status | Toxicity status | Allergenicity status |
---|---|---|---|---|
SLKKGSYPL | 1.3734 | Stable | Non- toxic | Non-allergic |
KIKTVSSEL | 1.4693 | Stable | Non- toxic | Non-allergic |
GNPCMKEKL | 0.0854 | Stable | Non- toxic | Non-allergic |
TMAGIAMTV | 1.9979 | Stable | Non- toxic | Non-allergic |
NCIDWVHKL | 0.6161 | Stable | Non- toxic | Non-allergic |
RAPNLVSYK | 0.1302 | Stable | Non- toxic | Non-Allergic |
RQMTGASLK | 1.7603 | Stable | Non- toxic | Non-allergic |
EVVPFAVFK | 0.4533 | Stable | Non- toxic | Non-allergic |
IQVSGVWKK | 1.5642 | Stable | Non- toxic | Non-allergic |
SAHGNPCMK | 0.9450 | Stable | Non- toxic | Non-allergic |
RVADNINQV | 0.5002 | Stable | Non- toxic | Non-allergic |
VVFAEDPHL | 1.4568 | Stable | Non- toxic | Non-allergic |
ITSTGTGTL | 0.0479 | Stable | Non- toxic | Non-allergic |
LSAKPIQRV | 0.1587 | Stable | Non- toxic | Non-allergic |
FKNSKKVYL | 1.5282 | Stable | Non- toxic | Non-allergic |
LKIAPRKVL | 0.6219 | Stable | Non- toxic | Non-allergic |
VQADLTLMF | 1.8723 | Stable | Non- toxic | Non-allergic |
HKGQYKGTM | 0.6591 | Stable | Non- toxic | Non-allergic |
TGFKISSAV | 1.6721 | Stable | Non- toxic | Non-allergic |
TKDQCQILH | 0.5748 | Stable | Non- toxic | Non-allergic |
VQADLTLM | 1.8470 | Stable | Non- toxic | Non-allergic |
GDIGVHMA | 0.2486 | Stable | Non- toxic | Non-allergic |
TKDQCQIL | 0.4858 | Stable | Non- toxic | Non-allergic |
LEKGKFPL | 1.6611 | Stable | Non- toxic | Non-allergic |
RETMAGIA | 0.3986 | Stable | Non- toxic | Non-allergic |
LKIAPRKV | 0.2515 | Stable | Non- toxic | Non-allergic |
RQMTGASL | 1.5040 | Stable | Non- toxic | Non-allergic |
Peptides | Antigenicity status | Allergenicity status | Toxicity status | Stability status |
---|---|---|---|---|
QSAHYLNNDGKMASV | Antigenic | Non-allergic | Non-toxic | Stable |
FLKIKTVSSELSCRE | Antigenic | Non-allergic | Non-toxic | Stable |
VLKCLKIAPRKVLNP | Antigenic | Non-allergic | Non-toxic | Stable |
No. | Predicted discontinuous epitope | Number of residues | Score |
---|---|---|---|
1. | A:V152, A:L153, A:N154, A:P155, A:G156, A:P157, A:G158, A:P159, A:G160, A:S161, A:K162, A:L163, A:T165, A:K166, A:K168 | 15 | 0.773 |
2. | A:K194, A:T195, A:E196, A:E197, A:N198, A:L199, A:L200, A:P201, A:D202 | 9 | 0.733 |
3. | A:D1, A:F2, A:A3, A:S4, A:C5, A:H6, A:T7, A:N8, A:G9, A:G10, A:I11, A:C12, A:L13, A:P14, A:N15, A:R16, A:C17, A:P18, A:G19, A:H20, A:G25, A:I26, A:C27, A:F28, A:R29, A:P30, A:R31, A:V32, A:K33, A:C34 | 30 | 0.711 |
4. | A:K180, A:G181, A:P182, A:G183, A:P184, A:G185, A:V186, A:Y187 | 8 | 0.697 |
5. | A:P117, A:G118, A:P119, A:G120, A:F121, A:E135, A:G136, A:P137, A:G138, A:P139, A:G140, A:V141, A:L142, A:K143, A:C144, A:L145, A:K146 | 17 | 0.669 |
6. | A:G81, A:T82, A:G83, A:T84, A:L85, A:A86, A:A87, A:Y88, A:R89, A:Q90, A:M91, A:T92, A:G93, A:A94, A:S95, A:K97, A:A98 | 17 | 0.639 |
7. | A:K125, A:T126, A:V127, A:S128, A:S129, A:E130 | 6 | 0.633 |
Toll-like Receptor | Docking score | Confidence Score | Ligand rmsd (Å) |
---|---|---|---|
TLR-7 | -278.26 | 0.9286 | 46.14 |
TLR-8 | -324.28 | 0.9703 | 94.62 |
Results
Antigenicity Prediction
Table 1 shows the antigenicity of selected proteins. The glycoprotein (M) sequences were seen to be the most antigenic of all the RVFV proteins.
Membrane Topology of the Selected Sequences
The development of subunit multi-epitope vaccines requires in-depth knowledge of transmembrane topology. To develop subunit multi-epitope vaccines, epitopes located in the extra-cytoplasmic segments are crucial for efficacy38. In this study, the TMHMM tool was used to analyze the topology of the different genome segments. The transmembrane topology of the selected proteins is presented in Table 2. A large part of the glycoproteins was in the extra-cytoplasmic portion, indicating their usability in designing a vaccine against RVFV.
Sequence Retrieval
A total of 60 RVFV M segment sequences from cattle, sheep, and goats were obtained from the NCBI. The sequences were obtained from a total of eight countries, including Kenya, Zimbabwe, South Africa, Egypt, Madagascar, and Namibia.
Multiple sequence alignment (MEGA)
The multiple sequence alignment showed large overlapping and conserved portions (Figure 1).
Prediction of Linear B-Cell Epitopes
Epitopes with between 10 and 20 amino acids that cut across at least two of the servers used (BepiPred, ABCpred, and SVMTriP) were selected. The physicochemical properties of the selected epitopes were then assessed for suitability in a vaccine construct. The results are presented in Table 3.
Prediction of CTLs
A total of 130 strongly binding CTL peptides were predicted for the selected BoLA alleles. These peptides were selected based on their binding score or ability. Strongly binding CTL peptides were examined for their stability index, toxicity, allergenicity, and antigenicity. Table 4 lists the 27 epitopes from the glycoprotein that passed these tests. However, not all the 27 made the final construct.
HTL Prediction
A total of 97 peptides demonstrated strong binding for the selected MHCII alleles and were further assessed to induce interleukin-4 and interleukin-10 cytokines. After being evaluated for their physicochemical characteristics, epitopes that showed the potential to stimulate the production of the aforementioned cytokines were also tested for antigenicity, allergenicity, and toxicity. Table 5 shows the HTL epitopes that scaled through the screening processes.
Primary Construct Assembly
The vaccine primary construct comprised a Bos taurus-specific beta defensin adjuvant, six CTL peptides, three HTL peptides, and two B-cell-based peptides. The adjuvant was attached to the first CTL peptide using the EAAAK linker and to subsequent CTL peptides using the AAY linker. The rest of the primary construct was linked using GPGPG linkers. Our final vaccine construct was a 202-amino-acid-long primary sequence (Figure 2).
Prediction of the Physicochemical Properties of the RVFV Vaccine
The antigenicity index for a chimeric model with 0.4 points was determined using VaxiJen v2.0 server output and found to be quite high (0.6926). The multi-epitope protein construct was also anticipated to be dispersible upon overexpression using Protein Sol. The 202-amino-acid protein had a theoretical isoelectric point (Pl) of 9.44 and a relative molar mass (Mr) of 21.4 kDa as per the ExPasy Protparam web server. The sequence also had 26 cations (Arg + Lys) and 12 anions (Asp + Glu) residues. The assembled sequence was also shown to be sturdy, with a stability index of 20.37. The multi-epitope had a DIVIDEND score of −0.121 along with an acyclic index of 81.19, which indicated that it was hydrophilic (Figure 3) and thermo-stable.
Codon Optimization
The JCAT server, reverse translation, and codon optimization (ECAI) optimizations for the adaptation of E. coli strain K-12 sub-strain MG1655 were performed35. The improved GC content after codon optimization was 53.3% at a Codon Adaptation Index (CAI) of 1.0. To successfully integrate the RVF construct into the E. coli expression system, a high GC content is a sign of stability. The restriction enzymes Xho1 and EcoR1 were utilized to clone the back-translated nucleotide into the E. coli expression system. Effective insertion of the segment into the pET28a (+) vector resulted in the production of a 6096 bp clone. The in silico cloning procedure of the vaccine in an Escherichia coli pET28a (+) vector is presented in Figure 4.
Prediction of Secondary and Tertiary Vaccine Structure
The SOPMA server was used to analyze the secondary structure of the intended protein, and the findings were as follows: alpha helix = 31.55%, random coil = 44.92%, beta turn = 5.35%, and extended strand = 18.18%. The tertiary structure is presented in Figure 5a.
Tertiary Structure Refinement and Validation
The PROCHECK assessment of the stoichiometric quality of the structure revealed the residue and overall structural computation. Ramachandran plot analysis indicated that 95.7 % of the protein remains were located in their preferred regions (Figure 5 c). Furthermore, results from ProSA, which predicts implied oversights of a 3D model, protein, predicted a negative score of −4.85, which is a favorable indicator of model quality (Figure 5 b).
Prediction of Discontinuous Epitopes
The ElliPro server predicted an aggregate of 91 conformational epitopes from 187 amino acid residues on the vaccine tertiary construct31. Moreover, the Jmol viewer allows for the uptake and foresight of immune epitopes in a given protein sequence or structure. Conformational epitopes with protrusion index scores greater than 0.7 are shown in Figure 5 a-c.
Molecular Docking
HDOCK predicted 10 models docking between the vaccine construct and TLR7. The rankings were based on the Docking Score, Confidence Score, and Ligand RMSD (Å) (Figure 7 and Table 7).
Immune Response Incentive
The server C-ImmSim simulates immune findings indicated in a system of effective immune systems and an extended duration of the vaccine candidate. The vaccine design, as shown in Figure 8, produced a strong and consistent main and secondary immune response. The main immune response was defined by the level of the IgM antibodies after 4–5 days of antigenic exposure. The basic immune response was distinguished by enhanced B-cell generation and increased IgM and IgG1 + IgG2 expression and IgM + IgG antibodies. The advanced vaccine construct not only evoked a strong B-cell increase but also led to a multitude of memory B-cells. Specific interleukin concentration, a high level of IFN-γ for a long interval, CD4 activity, HTL count, and B-lymphocyte count were revealed.
Discussion
It is important to note that the approach we took in this study is novel and different from the conventional approach already adopted for vaccine development for RVFV, with its aforementioned limitations, resulting in the construction of a safe, non-allergenic, and non-toxic subunit vaccine design that is capable of eliciting optimal immunity against RVF in ruminants and can be further developed into a vaccine for evaluation in ruminants.
RVF is a zoonotic illness spread by mosquitoes. It poses a lethal threat to humans and livestock in many sub-Saharan countries, such as South Africa, Egypt, Madagascar, Mauritania, and Senegal. The disease has been documented in the Arabian Peninsula as well as in the Middle East and India39. In animals, the disease has a high fatality rate, where it causes epizootics of abortion and fetal death and high mortality in neonatal ruminants. The virus also causes human infections, although with a lower mortality rate. The Smithburn live attenuated RVFV variant has been extensively adopted in different countries for prevention; however, like many live attenuated vaccines, it faces challenges with safety due to its reversal to virulence, characterized by vaccine-induced teratogenesis, abortion, and fetal death. As a result, it is not recommended for usage in developing nations40. Furthermore, the vaccine faces a challenge due to the risk of genetic reassortment41. Therefore, novel approaches to designing safe and effective vaccines against this disease are needed.
Advances in Whole-Genome Sequencing (WGS) and proteomics have increased our understanding of viral proteins, which are being used in vaccine design via a reverse vaccinology approach. The use of peptide vaccines has emerged as an outstanding alternative to conventional approaches to vaccine design42. Subunit epitope-based vaccines provide an excellent alternative when considering safety, practicability, and cost-effectiveness. Moreover, the potency and immunogenicity of these vaccines can be boosted through the selected engineering of target epitopes43. By applying recent developments in immunoinformatics and computational technologies, scientists can selectively construct vaccines with minimal side effects and allergenicity44. Consequently, some vaccines have been designed against various pathogens such as SARS-CoV-2, Ebola, hepatitis C, and cholera by applying this approach45, 46, 47, 48. Our objective in this study was to design a safe, non-allergenic, and non-toxic subunit vaccine that can elicit optimal immunity against RVF in ruminants.
We selected the M glycoprotein gene after immunoinformatics analysis and an extensive review of the literature on RVF vaccines. The M segment was chosen due to its high antigenicity and the presence of large extra-cytoplasmic segments. Furthermore, experimental vaccines developed using this protein have yielded good results. In different studies, RVFV vaccines developed using the glycoprotein showed excellent immune responses and demonstrated full protection against experimental heterologous challenge infections in sheep and cattle49, 50. The vaccine was also shown to differentiate naturally infected from vaccinated animals (DIVA)51, 52; in an immunological study, the pivotal role of the Gn and Gc segments in antibody production and the immune response against RVFV were also demonstrated. Furthermore, the M segments constitute surface proteins, so they have a great potential for inducing the production of neutralizing antibodies53, 54.
The glycoprotein gene was screened for linear B-cells, CD4 HTLs, and CD8 CTLs, which were all integrated into the eventual vaccine construct. Research by Dodd et al.55 has shown the role B-cells and CD4 HTLs play in mediating viral clearance. Specifically, CD4 cells were shown to play major roles in maintaining robust IgG and neutralizing antibody responses, as well as controlling viral replication in vivo. CD4 activates B-cells and signals isotype class switching, affinity maturation, and antibody production through its effect on antibody-mediated immunity. It also mediates cytotoxicity by stimulating CD8 cells55.
The final vaccine construct was made up of 11 epitopes, comprising six CTL peptides, three HTL peptides, and two linear B-cell peptides. All peptides had their physicochemical properties assessed to ensure that they were antigenic, non-allergenic, and non-toxic. The HTL peptides were also evaluated for their ability to induce IL-4 and IL-10. These peptides were linked with AAY and GPGPG linkers. Linkers are vital in vaccine design as they ensure that protein amino acid residues are situated in a conformation similar to their natural state. A 38-amino-acid-long Bos taurus beta-defensin was further added to increase the vaccine’s immunogenicity with the help of a rigid EAAAK linker.
Analysis of the vaccine’s antigenicity, allergenicity, and other physicochemical properties showed favorable results. The vaccine was found to be antigenic, non-allergenic, and non-toxic, indicating a favorable safety potential. Further physicochemical analysis indicated the vaccine to be stable, basic, and soluble.
The potency of the vaccine to induce an immune response was further evaluated by molecular docking analysis and immune simulation. Two toll-like receptors, TLR7 and TLR8, which have an affinity for viral RNA ligands, were selected. The vaccine construct had more binding affinity for TLR8, with a binding score of −324 than for TLR7, which had a binding score of −278. Furthermore, immune simulation with C-ImmSim showed a sustained immune response for up to 350 days after injection with 100 units. After 350 days, the IgG + IgM had dropped to a basal level of about 5000 antibody titers from a peak of about 30,000 that had been reached during the first 30 days of administration. There are three stages of immunity, which are innate or natural, adaptive or active, and passive immunity. Innate is a sort of general protection, passive immunity is a temporary immunity that lasts for a short time, and adaptive immunity develops throughout our lives, beginning when we are exposed to diseases or immunized against them. RVF is an evolving arboviral disease that requires concerted efforts for prevention and control. To this end, novel vaccines that can combine potency and safety are needed, both in animals and humans.
CONCLUSION
The vaccine construct designed in this study potentially possesses properties differing from those of conventional vaccines against RVF because the approach was different. The construct is safe, non-allergenic, and non-toxic, capable of eliciting optimal immunity against RVF in ruminants, and can be further developed into a vaccine for evaluation in ruminants.
In this study, we presented an immunoinformatics approach to developing a multi-epitope subunit RVFV vaccine for use in ruminants. The M glycoprotein gene was selected because of its high antigenicity and the availability of experimental evidence of its efficacy in vaccines. Six CTL, three HTL, and two B-cell epitopes were joined together in a chimeric construct and subjected to analysis. In-silico analysis demonstrated the immunogenicity and safety of the vaccine construct; however, further in vitro and in vivo analyses are required to validate the study.
Abbreviations
AAY: A type of linker used in the vaccine construct, ANN: Artificial Neural Networks, BA: Binding Affinity, BoLA: Bovine Leukocyte Antigen, CAI: Codon Adaptation Index, CTL: Cytotoxic T Lymphocytes, DIVA: Differentiating Infected from Vaccinated Animals, DRB1: HLA-DR beta-1, E. coli: Escherichia coli, EAAAK: A type of linker used in the vaccine construct, ExPASy: Expert Protein Analysis System, GC: Guanine-Cytosine, GPGPG: A type of linker used in the vaccine construct, HTL: Helper T Lymphocytes, IEDB: Immune Epitope Database, IL: Interleukin, JCAT: Java Codon Adaptation Tool, MEGA: Molecular Evolutionary Genetics Analysis, MHC: Major Histocompatibility Complex, NCBI: National Center for Biotechnology Information, pET-28a (+): A type of expression vector, ProSA-web: Protein Structure Analysis-web, RVF: Rift Valley Fever, RVFV: Rift Valley Fever Virus, SOPMA: Self-Optimized Prediction Method with Alignment, TLR: Toll-Like Receptor, TMHMM: Transmembrane Hidden Markov Model, WGS: Whole Genome Sequencing
Acknowledgments
None
Author’s contributions
Conceptualization and experimental design: EKO; data collection and analysis, ORT, FOT, APT, AMB, OIO, BVO, JIT; supervision and result interpretation: EKO, ORT; manuscript draft: EKO, ORT, IOA; formal analysis, review and editing: IOA. All authors commented on the previous versions of the manuscript, and read and approved the final manuscript.
Funding
The authors declare that they received no funds, grants, or other support during the preparation of this manuscript.
Availability of data and materials
Data and materials used and/or analyzed during the current study are available from the corresponding author on reasonable request.
Ethics approval and consent to participate
Not applicable.
Consent for publication
Not applicable.
Competing interests
The authors declare that they have no competing interests.
References
-
World Bank. People Pathogens and Our Planet: The Economics of One Health World Bank. Washington DC, 2012. . 2012
.
-
Centers for Disease Control and Prevention. Viral Special Pathogens Branch (VSPB). Available from: https://wwwcdcgov/vhf/rvf/abouthtml Accessed October 21 2023.
.
-
Paweska
J.T.,
Vuren
P.J. van,
The Role of Animals in Emerging Viral Diseases. Academic Press.
2014;
:
169-200
.
View Article Google Scholar -
Ferron
F.,
Weber
F.,
Torre
J.C. de la,
Reguera
J.,
Transcription and replication mechanisms of Bunyaviridae and Arenaviridae L proteins. Virus Research.
2017;
234
:
118-34
.
View Article PubMed Google Scholar -
Anyamba
A.,
Linthicum
K.J.,
Small
J.,
Britch
S.C.,
Pak
E.,
Rocque
S. de La,
Prediction, assessment of the Rift Valley fever activity in East and Southern Africa 2006-2008 and possible vector control strategies. The American Journal of Tropical Medicine and Hygiene.
2010;
83
(2)
:
43-51
.
View Article PubMed Google Scholar -
Rostala
M.K.,
Rossa
N.,
Machalabaa
C.,
Cordelb
C.,
Paweskac
J.T.,
Karesha
W.B.,
Benefits of a one health approach: an example using Rift Valley fever. One Health.
2018;
5
:
34-36
.
View Article Google Scholar -
The FAO-OIE-WHO collaboration: sharing responsibilities and coordinating global activities to address Health risks at the animal-human ecosystems interfaces - a tripartite concept note 2010 Available from:https://wwwwhoint/publications/m/item/the-fao-oie-who-collaboration Accessed October 21 2023. 2010
.
-
Daubney
R.,
Hudson
J.R.,
Garnham
P.C.,
Enzootic hepatitis or Rift Valley fever an undescribed virus disease of sheep cattle and man from East Africa. The Journal of Pathology and Bacteriology.
1931;
34
(4)
:
545-79
.
View Article Google Scholar -
Kitandwe
P.K.,
McKay
P.F.,
Kaleebu
P.,
Shattock
R.J.,
An overview of Rift Valley fever vaccine development strategies. Vaccines.
2022;
10
(11)
:
1794
.
View Article PubMed Google Scholar -
Luo
Z.,
Fu
J.,
Li
N.,
Liu
Z.,
Qin
T.,
Zhang
X.,
Immunogenic proteins and their vaccine development potential evaluation in outer membrane proteins (OMPs) of Flavobacterium columnare. Aquaculture and Fisheries.
2016;
1
:
11-8
.
View Article Google Scholar -
Doytchinova
I.A.,
Flower
D.R.,
VaxiJen: a server for prediction of protective antigens, tumour antigens and subunit vaccines. BMC Bioinformatics.
2007;
8
(1)
:
4
.
View Article PubMed Google Scholar -
Hallgren
J.,
Tsirigos
K.D.,
Pedersen
M.D.,
Armenteros
J.J. Almagro,
Marcatili
P.,
Nielsen
H.,
DeepTMHMM predicts alpha and beta transmembrane proteins using deep neural networks. BioRxiv.
2022
.
View Article Google Scholar -
Tamura
K.,
Stecher
G.,
Kumar
S.,
Kumar S. MEGA11: molecular evolutionary genetics analysis version 11. Molecular Biology and Evolution.
2021;
38
(7)
:
3022-7
.
View Article PubMed Google Scholar -
Edgar
R.C.,
MUSCLE: multiple sequence alignment with high accuracy and high throughput. Nucleic Acids Research.
2004;
32
(5)
:
1792-7
.
View Article PubMed Google Scholar -
Saha
S.,
Raghava
G.P.,
Prediction of continuous B-cell epitopes in an antigen using recurrent neural network. Proteins: Structure, Function, and Bioinformatics.
2006;
65
(1)
:
40-8
.
View Article Google Scholar -
Yao
B.,
Zhang
L.,
Liang
S.,
Zhang
C.,
SVMTriP: a method to predict antigenic epitopes using support vector machine to integrate tri-peptide similarity and propensity. PLoS One.
2012;
7
(9)
.
View Article PubMed Google Scholar -
Larsen
J.E.,
Lund
O.,
Nielsen
M.,
Improved method for predicting linear B-cell epitopes. Immunome Research.
2006;
2
(1)
:
2
.
View Article PubMed Google Scholar -
Walker
J.M.,
Rapley
M.,
Medical Biomethods Handbook Humana Press Totowa NJ USA, 2005. 2005
.
-
Gupta
S.,
Kapoor
P.,
Chaudhary
K.,
Gautam
A.,
Kumar
R.,
Raghava
G.P.,
Consortium
undefined Open Source Drug Discovery,
In silico approach for predicting toxicity of peptides and proteins. PLoS One.
2013;
8
(9)
.
View Article PubMed Google Scholar -
Dimitrov
I.,
Bangov
I.,
Flower
D.R.,
Doytchinova
I.,
AllerTOP v.2\textemdasha server for in silico prediction of allergens. Journal of Molecular Modeling.
2014;
20
(6)
:
2278
.
View Article PubMed Google Scholar -
Reynisson
B.,
Barra
C.,
Kaabinejadian
S.,
Hildebrand
W.H.,
Peters
B.,
Nielsen
M.,
Improved prediction of MHC II antigen presentation through integration and motif deconvolution of mass spectrometry MHC eluted ligand data. Journal of Proteome Research.
2020;
19
(6)
:
2304-15
.
View Article PubMed Google Scholar -
Jensen
K.K.,
Andreatta
M.,
Marcatili
P.,
Buus
S.,
Greenbaum
J.A.,
Yan
Z.,
Improved methods for predicting peptide binding affinity to MHC class II molecules. Immunology.
2018;
154
(3)
:
394-406
.
View Article PubMed Google Scholar -
Dhanda
S.K.,
Gupta
S.,
Vir
P.,
Raghava
G.P.,
Prediction of IL4 inducing peptides. Clinical & Developmental Immunology.
2013;
2013
.
View Article PubMed Google Scholar -
Nagpal
G.,
Usmani
S.S.,
Dhanda
S.K.,
Kaur
H.,
Singh
S.,
Sharma
M.,
Computer-aided designing of immunosuppressive peptides based on IL-10 inducing potential. Scientific Reports.
2017;
7
(1)
:
42851
.
View Article PubMed Google Scholar -
Bui
H.H.,
Sidney
J.,
Li
W.,
Fusseder
N.,
Sette
A.,
Development of an epitope conservancy analysis tool to facilitate the design of epitope-based diagnostics and vaccines. BMC Bioinformatics.
2007;
8
(1)
:
361
.
View Article PubMed Google Scholar -
Kavoosi
M.,
Creagh
A.L.,
Kilburn
D.G.,
Haynes
C.A.,
Strategy for selecting and characterizing linker peptides for CBM9-tagged fusion proteins expressed in Escherichia coli. Biotechnology and Bioengineering.
2007;
98
(3)
:
599-610
.
View Article PubMed Google Scholar -
Ali
M.,
Pandey
R.K.,
Khatoon
N.,
Narula
A.,
Mishra
A.,
Prajapati
V.K.,
Exploring dengue genome to construct a multi-epitope based subunit vaccine by utilizing immunoinformatics approach to battle against dengue infection. Scientific Reports.
2017;
7
(1)
:
9232
.
View Article PubMed Google Scholar -
Hebditch
M.,
Carballo-Amador
M.A.,
Charonis
S.,
Curtis
R.,
Warwicker
J.,
Protein-Sol: a web tool for predicting protein solubility from sequence. Bioinformatics (Oxford, England).
2017;
33
(19)
:
3098-100
.
View Article PubMed Google Scholar -
Geourjon
C.,
Deléage
G.,
SOPMA: significant improvements in protein secondary structure prediction by consensus prediction from multiple alignments. Computer Applications in the Biosciences.
1995;
11
(6)
:
681-4
.
View Article PubMed Google Scholar -
Jumper
J.,
Evans
R.,
Pritzel
A.,
Green
T.,
Figurnov
M.,
Ronneberger
O.,
Highly accurate protein structure prediction with AlphaFold. Nature.
2021;
596
(7873)
:
583-9
.
View Article PubMed Google Scholar -
Ponomarenko
J.,
Bui
H.H.,
Li
W.,
Fusseder
N.,
Bourne
P.E.,
Sette
A.,
ElliPro: a new structure-based tool for the prediction of antibody epitopes. BMC Bioinformatics.
2008;
9
(1)
:
514
.
View Article PubMed Google Scholar -
Heo
L.,
Park
H.,
Seok
C.,
GalaxyRefine: protein structure refinement driven by side-chain repacking. Nucleic Acids Research.
2013;
41
(Web Server issue)
.
View Article PubMed Google Scholar -
R. Laskowski,
M. MacArthur,
J. Thornton,
PROCHECK: Validation of protein-structure coordinates. International Tables for Crystallography.
2006;
Vol. F. ch. 25.2
:
722-725
.
-
Wiederstein
M.,
Sippl
M.J.,
ProSA-web: interactive web service for the recognition of errors in three-dimensional structures of proteins. Nucleic Acids Research.
2007;
35
(Web Server issue)
.
View Article PubMed Google Scholar -
Grote
A.,
Hiller
K.,
Scheer
M.,
Münch
R.,
Nörtemann
B.,
Hempel
D.C.,
JCat: a novel tool to adapt codon usage of a target gene to its potential expression host. Nucleic Acids Research.
2005;
33
(Web Server issue)
.
View Article PubMed Google Scholar -
Asghari
A.,
Nourmohammadi
H.,
Majidiani
H.,
Shariatzadeh
A.,
Shams
M.,
Ontazeri
F.M.,
In silico analysis and prediction of immunogenic epitopes for pre-erythrocytic proteins of the deadly Plasmodium falciparum. Infection, Genetics and Evolution.
2021;
93
:
104985
.
View Article Google Scholar -
Yan
Y.,
Zhang
D.,
Zhou
P.,
Li
B.,
Huang
S.Y.,
HDOCK: a web server for protein-protein and protein-DNA/RNA docking based on a hybrid strategy. Nucleic Acids Research.
2017;
45
:
365-73
.
View Article PubMed Google Scholar -
Jardine
J.G.,
Ota
T.,
Sok
D.,
Pauthner
M.,
Kulp
D.W.,
Kalyuzhniy
O.,
HIV-1 VACCINES. Priming a broadly neutralizing antibody response to HIV-1 using a germline-targeting immunogen. Science.
2015;
349
(6244)
:
156-61
.
View Article PubMed Google Scholar -
Gerken
K.N.,
LaBeaud
A.D.,
Mandi
H.,
Jackson
M. L'Azou,
Breugelmans
J.G.,
King
C.H.,
Paving the way for human vaccination against Rift Valley fever virus: A systematic literature review of RVFV epidemiology from 1999 to 2021. PLoS Neglected Tropical Diseases.
2022;
16
(1)
.
View Article PubMed Google Scholar -
Botros
B.,
Omar
A.,
Elian
K.,
Mohamed
G.,
Soliman
A.,
Salib
A.,
Adverse response of non-indigenous cattle of European breeds to live attenuated Smithburn Rift Valley fever vaccine. Journal of Medical Virology.
2006;
78
(6)
:
787-91
.
View Article PubMed Google Scholar -
Sall
A.A.,
Zanotto
P.M.,
Sene
O.K.,
Zeller
H.G.,
Digoutte
J.P.,
Thiongane
Y.,
Genetic reassortment of Rift Valley fever virus in nature. Journal of Virology.
1999;
73
(10)
:
8196-200
.
View Article PubMed Google Scholar -
World Health Organization. Guide line for production and quality control of synthetic peptide vaccine. WHO Technical Report Series. 1999;No989. 1999
.
-
Shey
R.A.,
Ghogomu
S.M.,
Esoh
K.K.,
Nebangwa
N.D.,
Shintouo
C.M.,
Nongley
N.F.,
In-silico design of a multi-epitope vaccine candidate against onchocerciasis and related filarial diseases. Scientific Reports.
2019;
9
(1)
:
4409
.
View Article PubMed Google Scholar -
Seib
K.L.,
Zhao
X.,
Rappuoli
R.,
Developing vaccines in the era of genomics: a decade of reverse vaccinology. Clinical Microbiology and Infection.
2012;
18
:
109-16
.
View Article PubMed Google Scholar -
Dash
R.,
Das
R.,
Junaid
M.,
Akash
M.F.,
Islam
A.,
Hosen
S.Z.,
In silico-based vaccine design against Ebola virus glycoprotein. Advances and Applications in Bioinformatics and Chemistry : AABC.
2017;
10
:
11-28
.
View Article PubMed Google Scholar -
Ikram
A.,
Zaheer
T.,
Awan
F.M.,
Obaid
A.,
Naz
A.,
Hanif
R.,
Exploring NS3/4A, NS5A and NS5B proteins to design conserved subunit multi-epitope vaccine against HCV utilizing immunoinformatics approaches. Scientific Reports.
2018;
8
(1)
:
16107
.
View Article PubMed Google Scholar -
Kar
T.,
Narsaria
U.,
Basak
S.,
Deb
D.,
Castiglione
F.,
Mueller
D.M.,
A candidate multi-epitope vaccine against SARS-CoV-2. Scientific Reports.
2020;
10
(1)
:
10895
.
View Article PubMed Google Scholar -
Oladipo
E.K.,
Akindiya
O.E.,
Oluwasanya
G.J.,
Akanbi
G.M.,
Olufemi
S.E.,
Adediran
D.A.,
Bioinformatics analysis of structural protein to approach a vaccine candidate against Vibrio cholerae infection. Immunogenetics.
2022;
\textbullet\textbullet\textbullet
:
1-16
.
View Article PubMed Google Scholar -
Faburay
B.,
Wilson
W.C.,
Gaudreault
N.N.,
Davis
A.S.,
Shivanna
V.,
Bawa
B.,
A recombinant Rift Valley fever virus glycoprotein subunit vaccine confers full protection against Rift Valley fever challenge in sheep. Scientific Reports.
2016;
6
(1)
:
27719
.
View Article PubMed Google Scholar -
Wilson
W.C.,
Faburay
B.,
Trujillo
J.D.,
Ragan
I.,
Sunwoo
S.Y.,
Morozov
I.,
Preliminary evaluation of a recombinant Rift Valley fever virus glycoprotein subunit vaccine providing full protection against heterologous virulent challenge in cattle. Vaccines.
2021;
9
(7)
:
748
.
View Article PubMed Google Scholar -
Faburay
B.,
Lebedev
M.,
McVey
D.S.,
Wilson
W.,
Morozov
I.,
Young
A.,
A glycoprotein subunit vaccine elicits a strong Rift Valley fever virus neutralizing antibody response in sheep. Vector Borne and Zoonotic Diseases (Larchmont, N.Y.).
2014;
14
(10)
:
746-56
.
View Article PubMed Google Scholar -
Wright
D.,
Allen
E.R.,
Clark
M.H.,
Gitonga
J.N.,
Karanja
H.K.,
Hulswit
R.J.,
Naturally acquired Rift Valley fever virus neutralizing antibodies predominantly target the Gn glycoprotein. iScience.
2020;
23
(11)
.
View Article PubMed Google Scholar -
Lorenzo
G.,
Martín-Folgar
R.,
Hevia
E.,
Boshra
H.,
Brun
A.,
Protection against lethal Rift Valley fever virus (RVFV) infection in transgenic IFNAR(-/-) mice induced by different DNA vaccination regimens. Vaccine.
2010;
28
(17)
:
2937-44
.
View Article PubMed Google Scholar -
Doyle
J.D.,
Barbeau
D.J.,
Cartwright
H.N.,
McElroy
A.K.,
Immune correlates of protection following Rift Valley fever virus vaccination. NPJ Vaccines.
2022;
7
(1)
:
129
.
View Article PubMed Google Scholar -
Dodd
K.A.,
McElroy
A.K.,
Jones
M.E.,
Nichol
S.T.,
Spiropoulou
C.F.,
Rift Valley fever virus clearance and protection from neurologic disease are dependent on CD4+ T cell and virus-specific antibody responses. Journal of Virology.
2013;
87
(11)
:
6161-71
.
View Article PubMed Google Scholar
Comments
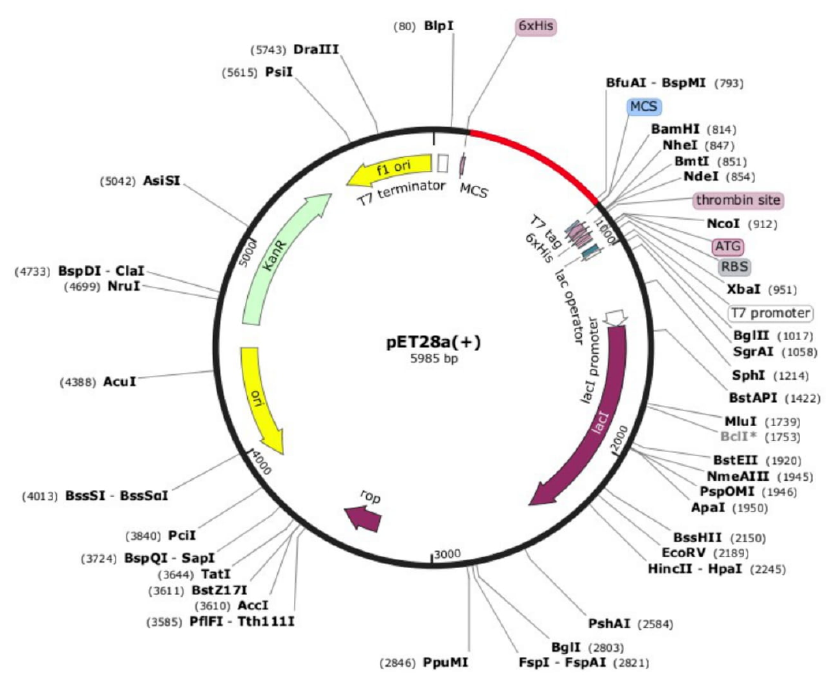
Article Details
Volume & Issue : Vol 11 No 2 (2024)
Page No.: 6233-6247
Published on: 2024-02-29
Citations
Copyrights & License

This work is licensed under a Creative Commons Attribution 4.0 International License.
Search Panel
- HTML viewed - 3498 times
- PDF downloaded - 1342 times
- XML downloaded - 94 times