Abstract
Introduction: Chromatin immunoprecipitation sequencing (ChIP-Seq) is a DNA sequencing technique for the identification of binding sites in genomic sequences. ChIP-Seq experiments are a combination of immunoprecipitation and sequencing techniques that are used for the identification of chromatin regions that bind molecules such as transcription factors (TFs), histones, and drugs. In this study, computational analysis of ChIP-Seq data was performed to predict the binding sites in breast cancer cells and their association with several molecules, such as TFs and drugs. A complete and comprehensive computational study has been performed to predict the binding sites of abemaciclib. Functional enrichment of selected motifs was performed to identify important motifs that function in breast cancer and show binding with the drug abemaciclib.
Methods and Materials: The ChIP-Seq analysis protocol was performed using the Galaxy server (https://usegalaxy.org/). The abemaciclib binding motif was identified using MEME tools. For this research, ChIP-Seq data from a breast cancer cell line was retrieved from the GEO database, accession number GSM4763932. This dataset includes ChIP-Seq of MCF-7 cells exposed to abemaciclib. The ENA browser was used to retrieve the data. Statistical analysis was performed using the default parameters of Fast-QC, Multi-QC, Map with BWA, Filter SAM and BAM, MACS2, and ChIP Seeker tools on the Galaxy server.
Results: Computational analysis identifies the abemaciclib consensus binding sequence as TGGCTCACGCCTGTAATCCCAGCACTTT, and this motif occurs 2980 times in the Homo sapiens reference genome hg19.
Conclusions: This study identifies the binding sites and affinity of abemaciclib in a breast cancer cell line.
Introduction
Cancer of the breast is uncontrolled production of breast cells1. Breast cancer is the second most common cancer diagnosed in women in the United States after skin cancer. Breast cancer may occur in both men and women, but it is much more prevalent in women. It is the most prevalent cause of death from cancer among women worldwide2. In more developed countries, incidence rates are high, while rates in less developed countries are low but rising rapidly.
Genomics studies have revealed genes associated with breast cancer. The identification and functional understanding of these genes are very important for the molecular diagnostics and drug development industries. Breast cancer genes such as BRCA1, BRCA2, CHEK2, ATM and PALB2 have been widely studied since these genes play a major role in breast cancer development3. Cyclin-dependent kinases 4 and 6 (CDK4/6) are pharmacological inhibitors and have a significant effect on the practice of oncology4. They are routinely recommended for estrogen receptor positive breast cancer therapy, and many studies are underway to assess their activity against other forms of cancer5. CDK4/6 mediates the cell cycle transition from G1 to S phase, and CDK4/6 inhibitors cause arrest of the G1 phase in tumor cells6. Other phenotypes in cancer cells could also be activated, including enhanced immunogenicity, apoptotic evasion, histologic tumor differentiation, and increased dependence on receptor tyrosine kinase signaling7. Abemaciclib is a CDK4- and CDK6-selective ATP-competitive, reversible kinase inhibitor that has demonstrated antitumor activity in clinical trials as a single agent in hormone receptor-positive (HR+) metastatic breast cancer8.
With advancements in next-generation sequencing technologies, genome-wide screening of binding sites can be performed by sequencing chromatin associated with proteins. Chromatin immunoprecipitation (ChIP-Seq) is used to identify the binding sites of proteins of interest at the genomic scale. ChIP-Seq analysis is used to identify the binding sequence for any drug or transcription factor9. Transcription factors are proteins that bind upstream of genes to enhance or initiate transcription10. Many genes are responsible for causing breast cancer, as described above11. As the main genes responsible for tumor formation in breast cancer are BRCA1 and BRCA2, ChIP-Seq can be used to identify other transcription factors that initiate the activation of BRCA1 or BRCA2 and facilitate the formation of tumors, causing cancer12.
ChIP-Seq is an effective technique for defining genome-wide DNA-binding sites for transcription factors and other proteins by combining chromatin immunoprecipitation (ChIP) with sequencing13. The DNA-bound protein is immunoprecipitated using a specific antibody14. Bound DNA is co-precipitated, purified, and sequenced. ChIP-Seq can be used to identify the binding sites of proteins associated with DNA and can be used to map a given protein's genome-wide binding sites15. Usually, ChIP-Seq begins with DNA–protein complex crosslinking. The samples are fragmented and processed to trim unbound oligonucleotides with an exonuclease. To immunoprecipitate a DNA–protein complex, protein-specific antibodies are used16. The DNA is extracted and sequenced, thereby identifying the sequences of the protein-binding sites with high resolution17. Insights into gene regulation events that play a role in different diseases and biological pathways, such as development and cancer progression, have been uncovered by the application of next-generation sequencing (NGS) to ChIP. ChIP-Seq allows the interactions between proteins and nucleic acids to be thoroughly studied on a genome-wide scale18.
Michigan Cancer Foundation-7 (MCF-7) is a breast cancer cell line that was first isolated from a 69-year-old Caucasian women in 197019. It is a well-known cell line used worldwide. It has features of mammary epithelial differentiation and is positive for many epithelial markers, such as β-catenin, cytokeratin 18 (CK18) and E-cadherin20. It is also negative signal for mesenchymal markers such as smooth muscle actin and vimentin21. MCF‑7 cells maintain the expression of other specific molecular markers of epithelium and do not express CD-44 ligand.
Abemaciclib is an antitumor agent and a dual cyclin-dependent kinase 4 (CDK4) and 6 (CDK6) inhibitor. CDK4/6 are involved in the cell cycle and in the event of unregulated activity, promote cancer development22. On September 28, 2017, the FDA approved abemaciclib for the treatment of HR-positive and HER2-negative advanced or metastatic breast cancer that has progressed following failed endocrine therapy, under the brand name Verzenio23. It is an oral inhibitor of cyclin-dependent kinase (CDK) that targets the cell cycle pathways of CDK4 (cyclin D1) and CDK6 (cyclin D3) with potential antineoplastic activity24. Abemaciclib inhibits CDK4 and 6 directly, thus inhibiting the phosphorylation of retinoblastoma (Rb) protein in early G125. Inhibition of Rb phosphorylation prevents the transition of the CDK-mediated G1-S process, thereby arresting the cell cycle in G1 phase, suppressing DNA synthesis and inhibiting the growth of cancer cells26. The interaction of abemaciclib with different genes such as AKT1, BARD1, and CDK4, and the functional consequences thereof, is shown in Table 1. Identification of binding sites for drugs is important to understand their function. Any mutation in the genome of the cell that disrupts a binding sites will result in loss of binding of the drug. In order for a drug to be effective in a mutated cell, such as a cancer cell, it is essential for the drug to bind its target sequence. Keeping this in mind, breast cancer data were used in the current study to identify the core binding sites in the genome of a breast cancer cell line. Functional analysis was also performed to identify functional sequences and core binding sites.
S.No | PubChem Gene | Interaction with Abemaciclib drug | Evidence PMID |
---|---|---|---|
1 | ABCB1 and ABCB2 | Abemaciclib results in decreasing the activity of ABCB1 and ABCB2 protein 27 | 27816545 |
2 | AKT1 | Abemaciclib results in decreased phosphorylation of and results in decreased activity of AKT1 protein 28 | 26909611 |
3 | BARD1 | Abemaciclib results in decreased expression of BARD1 mRNA 29 | 28620137 |
4 | CDK4 | Abemaciclib results in decreased activity of [CDK4 protein binds to CCND1 protein] 30 | 24919854 |
5 | RB1 | Abemaciclib results in decreased phosphorylation of RB1 protein 31 | 26909611 |
6 | SPARC | Abemaciclib results in increased expression of SPARC mRNA 32 | 28620137 |
7 | WNT4 | Abemaciclib results in decreased expression of WNT4 mRNA 33 | 28620137 |
Methods
Data Retrieval
The SRA (Short Read Archive) database was used for ChIP-Seq data retrieval. GSM4763932 ID was seen relevant because the study was on the MCF-7-cell line treated with abemaciclib. The SRA ID SRA1120692 was used. Two samples with accession numbers SRR12576544 and SRR12576545 were selected for motif enrichment and analysis. The ENA browser was used to retrieve the data (https://www.ebi.ac.uk/ena/browser/text-search?query=SRA1120692). The SRA IDs of MCF-7 DMSO and MCF-7 abemaciclib samples are shown in Table 2. FASTQ files were uploaded to the Galaxy server https://usegalaxy.org/ for analysis and annotation.
The Galaxy (https://usegalaxy.org/) web platform was used for the analysis and annotations of ChIP-Seq data. The methodology is shown in Figure 1. The tools used in Galaxy were Fast-QC, Multi-QC, Map with BWA, Filter SAM and BAM, MACS2, and ChIP Seeker. MEME Multiple EM for motif elicitation was used for motif identification at http://meme-suite.org/tools/meme. The steps followed for the identification of motifs are described below.
Step I: Fast-QC34
The Fast-QC tool was used to perform a quality check on raw sequence data. This offers a modular collection of tests that can be used to provide an indication of whether data have any issues that should be resolved before further processing. SRR12576544 and SRR12576545 were used as inputs for the Fast-QC analysis. The outputs were in HTML and .txt format. Fast‑QC tools produce statistical values that can be interpreted to identify filtering or preprocessing steps.
Step II: Multi-QC35
Multi-QC aggregates data from different samples into a single report. It compiles all results into a single HTML report that can be used for comparative study. The inputs for the Multi‑QC tool were the Fast-QC reports, and the compiled results were in the form of plots.
Step III: Map with BWA36
This tool is used to align short sequences to the large sequence database. SRR12576544 and SRR12576545 in fasta.qz file format were used as inputs for the map with BWA tool. The output was in BAM format, which can be visualized in the UCSC, bam.iobib.io and IGV genome browsers.
Step IV: Filter SAM or BAM37
This tool uses the samtools view commands in SAM Tools to filter SAM and BAM files on the mapping quality. This is a unique way of mapping by removing the non-required sequence. The input file is the result of the map with the bam tool, and the output is in BAM format.
Step V: Multi Bam Summary38
For two or more BAM files, multiBamSummary calculates the read coverage for genomic regions. The input was the result from the Filter SAM or BAM tool, and output was in the deeptools_coverage_matrix format. This result can be used for comparison of genomic alignments in different samples.
Step VI: plot Correlation39
This tool was used to analyze and visualize sample correlations based on multiBamSummary or multiBigwigSummary. The input was the result of multiBamSummary, and the output was in png format. This plot was used for the comparison of variations in sample files.
Step VII: plot fingerprint40
This tool samples indexed BAM files and graphs a cumulative read coverage profile for each file. The input was the same as for plot correlation, and the output was also in png format. This graph gives detailed information about the location of binding sites in genomic regions.
Step VIII: bamCoverage41
This method takes an alignment of reads or fragments as input (BAM file) and produces as output a coverage track (bigWig or bedGraph).
Step IX: MergeSamFiles42
Similar to the "merge" feature of Samtools. This tool is used to combine SAM or BAM files from separate runs and read groups into a single file.
Step X: Split 43
This is used to separate a combined file from a single BAM file. Aligned reads corresponding to the original four datasets are included in every subsequent BAM file.
Step XI: MACS244
ChIP-Seq model-based analysis (MACS) is a widely used method for identification of transcription factor binding sites. To determine the importance of enriched ChIP regions, the MACS algorithm captures the effect of genome complexity. It is also suitable for wider areas, although it was developed for the detection of transcription factor binding sites. The input was the result of Split, and the output was in the form of bed format for submit peaks and narrow peaks.
Step XII: ChIPSeeker45
ChIPseeker is a Bioconductor package in Galaxy for annotating ChIP-Seq data. The annotatePeak feature performs peak annotation. In addition to the distance from the peak to the TSS of its closest gene, the location and strand information of the nearest genes were recorded. The input file is obtained from the gencode tool, and the output is in the form of plots.
Step XIII: MEME-ChIP 46
MEME-ChIP performs motif analysis on large sets of sequences, such as those identified by ChIP-Seq experiments. The input was obtained from the Extract Genomic DNA tool, and the results were in HTML format.
Step XIV: plot Heatmap 47
This tool creates a heatmap for scores associated with genomic regions. This plot is used to visualize the genomic locations of predicted binding sites.
S.No | Name | SRR number | GSM number |
---|---|---|---|
1 | MCF-7 DMSO | SRR12576544 | GSM4763931 |
2 | MCF-7 Abemaciclib | SRR12576545 | GSM4763932 |
Sample Name | % Dups | % GC | M Seqs |
---|---|---|---|
SRR12576544_fastq_gz | 31.0% | 49% | 23.6 |
SRR12576545_fastq_gz | 8.0% | 46% | 21.8 |
Results
Following the protocol described in Figure 1, Fast-QC was performed for SRR12576544 and SRR12576545 samples in Galaxy, followed by Multi-QC. The input file used for this workflow was in fastq format, and the output file generated from Multi-QC was in HTML format. The results of Multi-QC are shown in Table 3. The comparison between sample files in terms of the number of duplicate reads, GC content and maximum length (M Seq) shows that both files can be used for further study. Figure 2 shows the number of duplicate and unique reads in both samples.
The BAM file was generated from the fastq.gz file by using Map with the BWA tool of Galaxy. The alignment between the sample file and the reference genome, hg19, was performed with the tool Map with BWA to identify the similarity between read sequences and genomic sequences. The results were in bam.iobio, IGV and UCSC formats, which were visualized using https://bam.iobio.io/home, Integrative Genomics Viewer (IGV) (https://software.broadinstitute.org/software/igv/) and the UCSC Genome Browser (https://genome.ucsc.edu/), respectively. The data used for this study satisfied all the parameters of quality control and were of very high quality, so the data were not trimmed or postprocessed in any way before mapping. Figure 3 and Figure 4 show the result of alignment of the read sequences with the hg19 reference genome. Figure 3 shows the alignment between the MCF-7 vehicle control reads and the reference human genome (SRR12576544 to hg19). Figure 4 shows the alignment between the MCF-7 abemaciclib sample and the reference genome (SRR12576545 to hg19). Both samples had more than 95% alignment with the reference genome.
All non-uniquely mapped reads were identified. This was accomplished by simply filtering out all reads with a mapping quality of less than 20 using NGS:SAMtools (SAM or BAM filter). Then, the mapping quality of the mapped reads was analyzed. The multiBamSummary tool was used to calculate the average read coverage for a list of two Bam files. Then, the plotCorrelation tool was used to create a heatmap of correlation scores between the samples, as shown in Figure 5, demonstrating that both files have approximately the same number of aligned regions.
plotFingerprint was used to identify the signal strength of the ChIP sample. Figure 6 shows the comparison of MCF-7 DMSO (SRR12576544, red) and MCF-7 abemaciclib (SRR12576545, green), which shows that there might be some variation in the aligned regions that can be explored in further studies.
The input for the MACS2 peak calling tool was obtained by merging the BAM file with the tool MergeSamFiles, and then the split tool was used to separate the merged file into the individual BAM files. Aligned reads corresponding to the original two datasets were included in each subsequent BAM file. Peak calling was performed using the MACS2 package, the results of which are shown in Figure 7. This tool finds the best parameters for the MACS2 peak calling.
The ChIPseeker tool was used to annotate the ChIP-Seq data. It annotates ChIP peaks and provides functionality to visualize the coverage of ChIP peaks over chromosomes and peak profiles that bind to TSS regions. ChIP peak profile comparison and annotation are also provided. In addition, it supports the evaluation of substantial overlap between ChIP-Seq datasets. Currently, ChIPseeker includes 17,000 GEO database bed file details. The input was given after performing the gene pool, which helped identify promoter, exon, intron, UTR, and downstream regions.
The output was in the form of plots and Excel files, which give all introns and exons with chromosome numbers. The result of the peak annotation is shown in Figure 8, which shows the percentage of identified peaks in promoter, exon, intron, and downstream regions. This is important to identify functional regions that show alignment with sample files. The reference genome result shows that there are alignment regions in almost all functional regions of the genome, such as promoters, genes, and exons.
De novo motif discovery, motif enrichment analysis, motif position analysis and motif clustering were performed using MEME-ChIP, providing a detailed image of the motifs that are enriched in the input sequences. Two complementary types of de novo motif discovery are performed by MEME-ChIP: weight matrix-based discovery for high accuracy and word-based discovery for high sensitivity. The study of motif enrichment using human, mouse, worm, fly and other model organism motifs offers even greater sensitivity.
The Extract Genomic DNA tool was used to generate genomic sequences corresponding to the ChIP peaks. Figure 9 shows the motif that was predicted using MEME ChIP, and Figure 10 shows the motif TGGCTCACGCCTGTAATCCCAGCACTTT, which was identified in 2980 positions in the Homo sapiens reference genome hg19.
A heatmap was generated to visualize the position of the motif in the whole genome (Figure 11). The blue region in the plot depicts the region of all motifs in the whole genome, showing the regions across the genome that represent motifs and binding sites. Heatmap results verify that abemaciclib has binding regions in this breast cancer cell line, and motifs identified are true motifs that have function in cells.
Discussion
ChIP-Seq is a next-generation sequencing technology that is used to identify binding sites genome-wide. This technique has been explored in the drug development industry to characterize the effects of drugs and identify conserved regions of their binding sites. Since drug binding site identification is important to study the functionality and effectiveness of drugs, ChIP-Seq is used to study novel drugs and their effectiveness. It can also be used to screen ligand libraries for binding efficiency in cancerous cells48. Studies of various diseases and cancers have identified variations in binding sites and differential binding sites for drugs49. ChIP-Seq technology has been explored in different dimensions to study breast cancer cells and to identify novel drugs that can stop breast cancer50. Studies have been carried out mainly to identify drugs against breast cancer51. In this study, computational analysis of ChIP‑Seq data of breast cancer cells was performed to identify binding sites of the drug abemaciclib. The binding motif for abemaciclib was identified as TGGCTCACGCCTGTAATCCCAGCACTTT. Mapping of the identified motif to the human reference genome shows that the predicted motif occurs in 2980 positions in the hg19 reference genome. The results identify the binding sites for abemaciclib in the MCF-7 genome. This study can be used to better understand the function of abemaciclib against breast cancer and provide insight into its conserved and functional region in the human genome.
Conclusions
Breast cancer is the most common type of cancer in women. Breast cancers can become metastatic when breast cancer cells spread to different organs of the body through blood vessels or nodes. Currently, abemaciclib is used to treat breast cancer, and studies have been performed to identify the role of abemaciclib in breast cancer. In this research, ChIP-Seq data were retrieved from the SRA database, and the interaction between abemaciclib and chromatin was studied. These data were selected to identify the motif that abemaciclib binds to, which can be explored in different dimensions to understand the function of the motif and the drug.It can be used in toxicology studies, or in studies of the effect of abemaciclib in treating breast cancer. Further verification and wet lab study are required to study the function of the identified motif and its interaction with abemaciclib.
Abbreviations
ChIP-Seq: Chromatin immunoprecipitation sequencing, TFs: transcription factors, MCF: Michigan Cancer Foundation-7, SRA: Short Read Archive, QC: Quality Control, SAM: Sequence Alignment Map, BAM: Binary Alignment Map, MACS2: Model-based Analysis of ChIP-Seq, MEME: Multiple Expectation maximizations for Motif Elicitation
Acknowledgments
We would like to acknowledge Amity Institute of biotechnology, Amity University Uttar Pradesh, Lucknow campus for providing us facilities to conducting this study. This research project is not funded by any specific grant from funding agencies in the public, commercial, or non-profit sectors.
Author’s contributions
Author Utkarsh Deep has done the work on methodology and interpretation of results and Ruchi Yadav contributed as framing methodology and analysis of the results. All authors read and approved the final manuscript.
Funding
None.
Availability of data and materials
Data and materials used and/or analyzed during the current study are available from the corresponding author on reasonable request.
Ethics approval and consent to participate
Not applicable.
Consent for publication
Not applicable.
Competing interests
The authors declare that they have no competing interests.
References
-
Vasconcelos
A.G.,
Valim
M.O.,
Amorim
A.G.,
Amaral
C.P. do,
Almeida
M.P. de,
Borges
T.K.,
Cytotoxic activity of poly-ɛ-caprolactone lipid-core nanocapsules loaded with lycopene-rich extract from red guava (Psidium guajava L.) on breast cancer cells. Food Research International.
2020;
136
:
109548
.
View Article PubMed Google Scholar -
Wu
C.,
Wei
Q.,
Utomo
V.,
Nadesan
P.,
Whetstone
H.,
Kandel
R.,
Side population cells isolated from mesenchymal neoplasms have tumor initiating potential. Cancer Research.
2007;
67
(17)
:
8216-22
.
View Article PubMed Google Scholar -
De Coppi
P.,
Callegari
A.,
Chiavegato
A.,
Gasparotto
L.,
Piccoli
M.,
Taiani
J.,
Amniotic fluid and bone marrow derived mesenchymal stem cells can be converted to smooth muscle cells in the cryo-injured rat bladder and prevent compensatory hypertrophy of surviving smooth muscle cells. The Journal of Urology.
2007;
177
(1)
:
369-76
.
View Article PubMed Google Scholar -
Singh-Ranger
G.,
Mokbel
K.,
Current concepts in cyclooxygenase inhibition in breast cancer. Journal of Clinical Pharmacy and Therapeutics.
2002;
27
(5)
:
321-7
.
View Article PubMed Google Scholar -
Goel
S.,
DeCristo
M.J.,
McAllister
S.S.,
Zhao
J.J.,
CDK4/6 inhibition in cancer: beyond cell cycle arrest. Trends in Cell Biology.
2018;
28
(11)
:
911-25
.
View Article PubMed Google Scholar -
Robert
M.,
Frenel
J.S.,
Bourbouloux
E.,
Rigaud
D.B.,
Patsouris
A.,
Augereau
P.,
An update on the clinical use of CDK4/6 inhibitors in breast Cancer. Drugs.
2018;
78
(13)
:
1353-62
.
View Article PubMed Google Scholar -
Sánchez-Martínez
C.,
Gelbert
L.M.,
Lallena
M.J.,
Dios
A. de,
Cyclin dependent kinase (CDK) inhibitors as anticancer drugs. Bioorganic & Medicinal Chemistry Letters.
2015;
25
(17)
:
3420-35
.
View Article PubMed Google Scholar -
Schaer
D.A.,
Beckmann
R.P.,
Dempsey
J.A.,
Huber
L.,
Forest
A.,
Amaladas
N.,
The CDK4/6 inhibitor abemaciclib induces a T cell inflamed tumor microenvironment and enhances the efficacy of PD-L1 checkpoint blockade. Cell Reports.
2018;
22
(11)
:
2978-94
.
View Article PubMed Google Scholar -
Harper
J.W.,
Elledge
S.J.,
Keyomarsi
K.,
Dynlacht
B.,
Tsai
L.H.,
Zhang
P.,
Inhibition of cyclin-dependent kinases by p21. Molecular Biology of the Cell.
1995;
6
(4)
:
387-400
.
View Article PubMed Google Scholar -
Wu
T.,
Chen
Z.,
To
K.K.,
Fang
X.,
Wang
F.,
Cheng
B.,
Effect of abemaciclib (LY2835219) on enhancement of chemotherapeutic agents in ABCB1 and ABCG2 overexpressing cells in vitro and in vivo. Biochemical Pharmacology.
2017;
124
:
29-42
.
View Article PubMed Google Scholar -
Chen
K.,
Jiao
X.,
Di Rocco
A.,
Shen
D.,
Xu
S.,
Ertel
A.,
Endogenous cyclin D1 promotes the rate of onset and magnitude of mitogenic signaling via Akt1 Ser473 phosphorylation. Cell Reports.
2020;
32
(11)
:
108151
.
View Article PubMed Google Scholar -
Knudsen
E.S.,
Hutcheson
J.,
Vail
P.,
Witkiewicz
A.K.,
Biological specificity of CDK4/6 inhibitors: dose response relationship, in vivo signaling, and composite response signature. Oncotarget.
2017;
8
(27)
:
43678-91
.
View Article PubMed Google Scholar -
Torres-Guzmán
R.,
Calsina
B.,
Hermoso
A.,
Baquero
C.,
Alvarez
B.,
Amat
J.,
Preclinical characterization of abemaciclib in hormone receptor positive breast cancer. Oncotarget.
2017;
8
(41)
:
69493-507
.
View Article PubMed Google Scholar -
Beck
T.N.,
Georgopoulos
R.,
Shagisultanova
E.I.,
Sarcu
D.,
Handorf
E.A.,
Dubyk
C.,
EGFR and RB1 as dual biomarkers in HPV-negative head and neck cancer. Molecular Cancer Therapeutics.
2016;
15
(10)
:
2486-97
.
View Article PubMed Google Scholar -
Jin
J.,
Fang
H.,
Yang
F.,
Ji
W.,
Guan
N.,
Sun
Z.,
Combined inhibition of ATR and WEE1 as a novel therapeutic strategy in triple-negative breast cancer. Neoplasia (New York, N.Y.).
2018;
20
(5)
:
478-88
.
View Article PubMed Google Scholar -
Testa
U.,
Castelli
G.,
Pelosi
E.,
Breast cancer: a molecularly heterogenous disease needing subtype-specific treatments. Medical Sciences : Open Access Journal.
2020;
8
(1)
:
18
.
View Article PubMed Google Scholar -
Kaufmann
K.,
Muiño
J.M.,
Osteras
M.,
Farinelli
L.,
Krajewski
P.,
Angenent
G.C.,
Chromatin immunoprecipitation (ChIP) of plant transcription factors followed by sequencing (ChIP-SEQ) or hybridization to whole genome arrays (ChIP-CHIP). Nature Protocols.
2010;
5
(3)
:
457-72
.
View Article PubMed Google Scholar -
Lee
T.I.,
Johnstone
S.E.,
Young
R.A.,
Chromatin immunoprecipitation and microarray-based analysis of protein location. Nature Protocols.
2006;
1
(2)
:
729-48
.
View Article PubMed Google Scholar -
Choul-Li
S.,
Legrand
A.J.,
Bidon
B.,
Vicogne
D.,
Villeret
V.,
Aumercier
M.,
Ets-1 interacts through a similar binding interface with Ku70 and Poly (ADP-Ribose) Polymerase-1. Bioscience, Biotechnology, and Biochemistry.
2018;
82
(10)
:
1753-9
.
View Article PubMed Google Scholar -
Buck
M.J.,
Lieb
J.D.,
ChIP-chip: considerations for the design, analysis, and application of genome-wide chromatin immunoprecipitation experiments. Genomics.
2004;
83
(3)
:
349-60
.
View Article PubMed Google Scholar -
Stanojević
D.,
Hoey
T.,
Levine
M.,
Sequence-specific DNA-binding activities of the gap proteins encoded by hunchback and Krüppel in Drosophila. Nature.
1989;
341
(6240)
:
331-5
.
View Article PubMed Google Scholar -
Kulski
J.K.,
Next-Generation Sequencing — An Overview of the History, Tools, and “Omic” Applications. In: Kulski, J. , editor. Next Generation Sequencing - Advances, Applications and Challenges [Internet]. London: IntechOpen; 2016 [cited 2022 Mar 31]..
.
View Article Google Scholar -
Su
Z.,
Ning
B.,
Fang
H.,
Hong
H.,
Perkins
R.,
Tong
W.,
Next-generation sequencing and its applications in molecular diagnostics. Expert Review of Molecular Diagnostics.
2011;
11
(3)
:
333-43
.
View Article PubMed Google Scholar -
Forde
B.M.,
O'Toole
P.W.,
Next-generation sequencing technologies and their impact on microbial genomics. Briefings in Functional Genomics.
2013;
12
(5)
:
440-53
.
View Article PubMed Google Scholar -
Olkhov-Mitsel
E.,
Bapat
B.,
Strategies for discovery and validation of methylated and hydroxymethylated DNA biomarkers. Cancer Medicine.
2012;
1
(2)
:
237-60
.
View Article PubMed Google Scholar -
Serrati
S.,
Summa
S. De,
Pilato
B.,
Petriella
D.,
Lacalamita
R.,
Tommasi
S.,
Next-generation sequencing: advances and applications in cancer diagnosis. OncoTargets and Therapy.
2016;
9
:
7355-65
.
View Article PubMed Google Scholar -
Fujioka
T.,
Kubota
K.,
Mori
M.,
Kikuchi
Y.,
Katsuta
L.,
Kasahara
M.,
Distinction between benign and malignant breast masses at breast ultrasound using deep learning method with convolutional neural network. Japanese Journal of Radiology.
2019;
37
(6)
:
466-72
.
View Article PubMed Google Scholar -
Freedman
D.M.,
Dosemeci
M.,
McGlynn
K.,
Sunlight and mortality from breast, ovarian, colon, prostate, and non-melanoma skin cancer: a composite death certificate based case-control study. Occupational and Environmental Medicine.
2002;
59
(4)
:
257-62
.
View Article PubMed Google Scholar -
Lee
A.J.,
Cunningham
A.P.,
Tischkowitz
M.,
Simard
J.,
Pharoah
P.D.,
Easton
D.F.,
Incorporating truncating variants in PALB2, CHEK2, and ATM into the BOADICEA breast cancer risk model. Genetics in Medicine.
2016;
18
(12)
:
1190-8
.
View Article PubMed Google Scholar -
Wildiers
H.,
de Glas
N.A.,
Anticancer drugs are not well tolerated in all older patients with cancer. The Lancet. Healthy Longevity.
2020;
1
(1)
:
e43-7
.
View Article Google Scholar -
Franco
J.,
Witkiewicz
A.K.,
Knudsen
E.S.,
CDK4/6 inhibitors have potent activity in combination with pathway selective therapeutic agents in models of pancreatic cancer. Oncotarget.
2014;
5
(15)
:
6512-25
.
View Article PubMed Google Scholar -
Mardis
E.R.,
Next-generation sequencing platforms. Annual Review of Analytical Chemistry (Palo Alto, Calif.).
2013;
6
(1)
:
287-303
.
View Article PubMed Google Scholar -
Spring
L.M.,
Wander
S.A.,
Andre
F.,
Moy
B.,
Turner
N.C.,
Bardia
A.,
Cyclin-dependent kinase 4 and 6 inhibitors for hormone receptor-positive breast cancer: past, present, and future. Lancet.
2020;
395
(10226)
:
817-27
.
View Article PubMed Google Scholar -
Nigro
A.,
Ricciardi
L.,
Salvato
I.,
Sabbatino
F.,
Vitale
M.,
Crescenzi
M.A.,
Enhanced expression of CD47 is associated with off-target resistance to tyrosine kinase inhibitor gefitinib in NSCLC. Frontiers in Immunology.
2020;
10
:
3135
.
View Article PubMed Google Scholar -
Dey
N.,
De
P.,
Leyland-Jones
B.,
PI3K-AKT-mTOR inhibitors in breast cancers: from tumor cell signaling to clinical trials. Pharmacology & Therapeutics.
2017;
175
:
91-106
.
View Article PubMed Google Scholar -
He
X.,
Chen
C.C.,
Hong
F.,
Fang
F.,
Sinha
S.,
Ng
H.H.,
A biophysical model for analysis of transcription factor interaction and binding site arrangement from genome-wide binding data. PLoS One.
2009;
4
(12)
:
e8155
.
View Article PubMed Google Scholar -
Sawadogo
M.,
Roeder
R.G.,
Interaction of a gene-specific transcription factor with the adenovirus major late promoter upstream of the TATA box region. Cell.
1985;
43
(1)
:
165-75
.
View Article PubMed Google Scholar -
Jatoi
I.,
Anderson
W.F.,
Jeong
J.H.,
Redmond
C.K.,
Breast cancer adjuvant therapy: time to consider its time-dependent effects. Journal of Clinical Oncology.
2011;
29
(17)
:
2301-4
.
View Article PubMed Google Scholar -
Hedenfalk
I.,
Ringnér
M.,
Ben-Dor
A.,
Yakhini
Z.,
Chen
Y.,
Chebil
G.,
Molecular classification of familial non-BRCA1/BRCA2 breast cancer. Proceedings of the National Academy of Sciences of the United States of America.
2003;
100
(5)
:
2532-7
.
View Article PubMed Google Scholar -
Zhang
W.,
Cui
H.,
Wong
L.J.,
Application of next generation sequencing to molecular diagnosis of inherited diseases. Top Curr Chem.
2014;
336
:
19-45
.
View Article Google Scholar -
Naidoo
N.,
Pawitan
Y.,
Soong
R.,
Cooper
D.N.,
Ku
C.S.,
Human genetics and genomics a decade after the release of the draft sequence of the human genome. Human Genomics.
2011;
5
(6)
:
577-622
.
View Article PubMed Google Scholar -
Ziogas
D.E.,
Genome-based approaches for the diagnosis of breast cancer: a review with perspective. Breast Cancer Management.
2014;
3
(2)
:
173-93
.
View Article Google Scholar -
Watt
A.C.,
Cejas
P.,
DeCristo
M.J.,
Metzger-Filho
O.,
Lam
E.Y.,
Qiu
X.,
CDK4/6 inhibition reprograms the breast cancer enhancer landscape by stimulating AP-1 transcriptional activity. Naturaliste Canadien.
2021;
2
(1)
:
34-48
.
View Article PubMed Google Scholar -
Newell
R.,
Pienaar
R.,
Balderson
B.,
Piper
M.,
Essebier
A.,
Bodén
M.,
ChIP-R: assembling reproducible sets of ChIP-seq and ATAC-seq peaks from multiple replicates. Genomics.
2021;
113
(4)
:
1855-66
.
View Article PubMed Google Scholar -
Han
Z.,
Yang
B.,
Wang
Q.,
Hu
Y.,
Wu
Y.,
Tian
Z.,
Comprehensive analysis of the transcriptome-wide m6A methylome in invasive malignant pleomorphic adenoma. Cancer Cell International.
2021;
21
(1)
:
142
.
View Article PubMed Google Scholar -
Yu
C.P.,
Kuo
C.H.,
Nelson
C.W.,
Chen
C.A.,
Soh
Z.T.,
Lin
J.J.,
Discovering unknown human and mouse transcription factor binding sites and their characteristics from ChIP-seq data.. Proceedings of the National Academy of Sciences.
2021;
118
(20)
:
e2026754118
.
View Article Google Scholar -
Kang
Y.,
Kang
J.,
Kim
Y.W.,
Kim
A.,
ChIP-seq Library Preparation and NGS Data Analysis Using the Galaxy Platform. Journal of Life Science.
2021;
31
:
410-7
.
View Article Google Scholar -
Li
Q.L.,
Lin
X.,
Yu
Y.L.,
Chen
L.,
Hu
Q.X.,
Chen
M.,
Genome-wide profiling in colorectal cancer identifies PHF19 and TBC1D16 as oncogenic super enhancers. Nature Communications.
2021;
12
(1)
:
6407
.
View Article PubMed Google Scholar -
Polit
L.,
Kerdivel
G.,
Gregoricchio
S.,
Esposito
M.,
Guillouf
C.,
Boeva
V.,
CHIPIN: ChIP-seq inter-sample normalization based on signal invariance across transcriptionally constant genes. BMC Bioinformatics.
2021;
22
(1)
:
407
.
View Article PubMed Google Scholar -
Qin
H.L.,
Wang
X.J.,
Yang
B.X.,
Du
B.,
Yun
X.L.,
Notoginsenoside R1 attenuates breast cancer progression by targeting CCND2 and YBX3. Chinese Medical Journal.
2021;
134
(5)
:
546-54
.
View Article PubMed Google Scholar -
Vishnubalaji
R.,
Alajez
N.M.,
Epigenetic regulation of triple negative breast cancer (TNBC) by TGF-β signaling. Scientific Reports.
2021;
11
(1)
:
15410
.
View Article PubMed Google Scholar
Comments
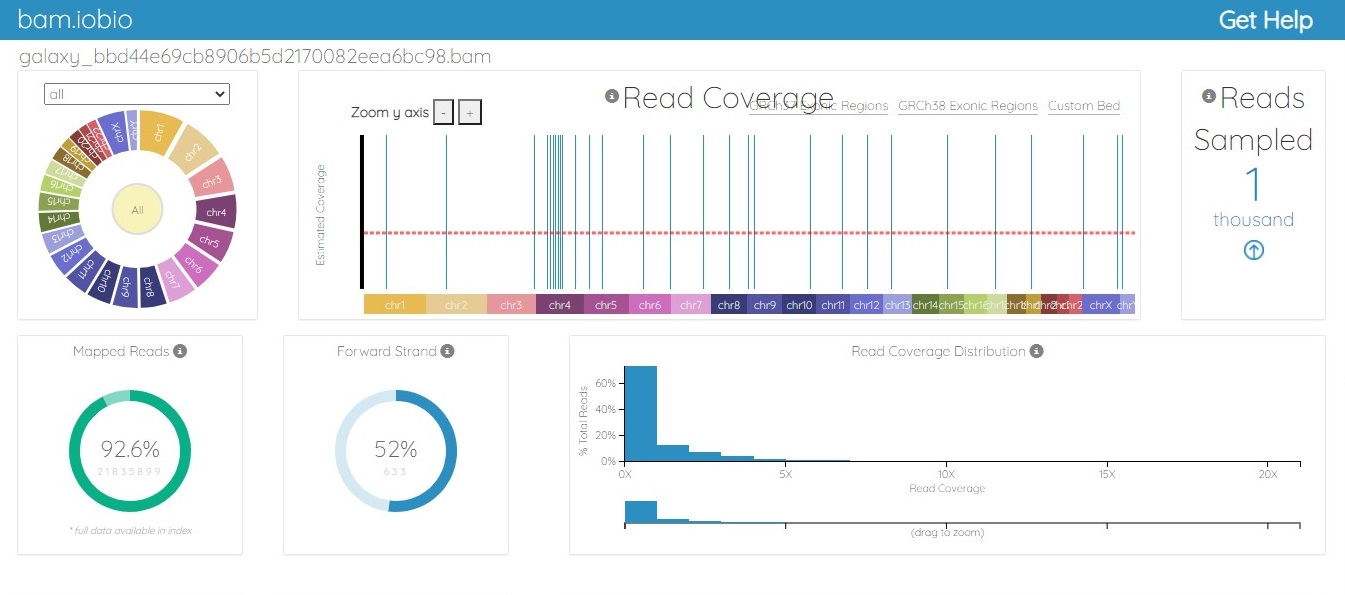
Article Details
Volume & Issue : Vol 9 No 3 (2022)
Page No.: 4971-4985
Published on: 2022-03-31
Citations
Copyrights & License

This work is licensed under a Creative Commons Attribution 4.0 International License.
Search Panel
- HTML viewed - 5076 times
- PDF downloaded - 1315 times
- XML downloaded - 0 times